
Woman, holding
(2020)
Steel, plastic, epoxy, printed image, wax, electronics, tablets, thread, fabric.
190cm x 260cm x 60cm
Part of a group show Strangers in a strange land
Curated by Unfinished Art Space
at MUŻA National Museum of Art, Valletta MT
The work addresses algorithmic bias in commercially available image description and text-to-image services. Presented in an ambiguous form, resembling a shrine, memorial, or futuristic display, the work delves into the biases inherent in commercial facial analysis and image description services trained on datasets like ImageNet and others.
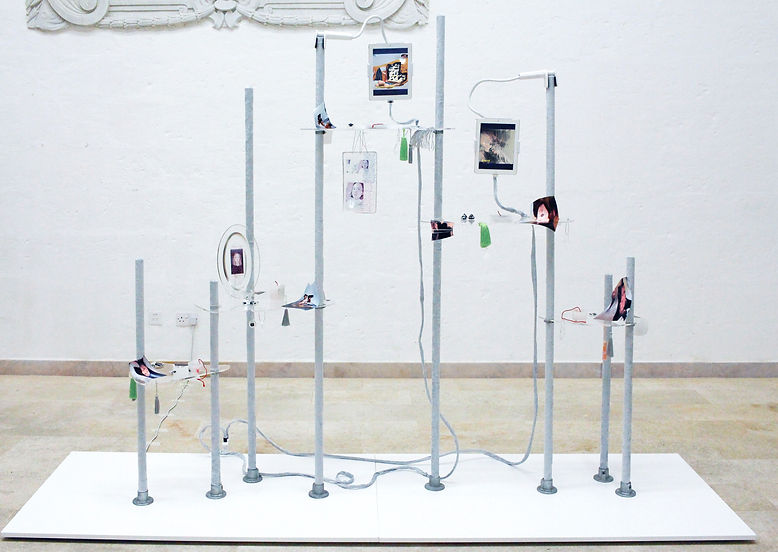
Woman, holding (2020)
installation view.

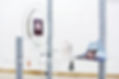
To create this work, multiple images of the artist were processed through various commercial text-to-image machine learning services and ML image descriptors. These services displayed little bias when describing non-human and male subjects, avoiding evaluative descriptors like 'pretty,' 'good looking,' or 'sexy' for nature, cityscapes, or clothed men. However, when describing women, they often employed evaluative descriptors. Shirtless male images were rarely described similarly, while images of women rarely escaped sexualized undertones. For instance, a shirtless man in a club ad was labelled as 'serious' and 'fine-looking.'
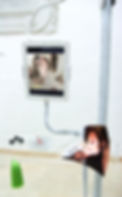

In a converse action using commercial text-to-image services, the text output from the image description service was processed. The artist removed evaluative descriptors like 'pretty' and 'good looking.' For example, an image description like 'woman in front of a mirror' generated a semi-abstract representation resembling a posed selfie in underwear, a beach photo, or a mirror selfie—all sharing a visual language of objectifying women.
While algorithms may seem neutral, they are ultimately created by people with their biases. The work's title, "woman, holding," derives from a frequently encountered image description, suggesting that the algorithms perceive women as caregivers. Descriptive algorithms rely on datasets tainted by biases from individuals (mostly men) who labelled images, shaping perceptions about potential criminals and gender roles in professions like doctors, lawyers, and scientists.

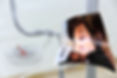
Woman, holding (2020)
video element
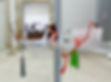


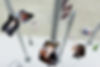
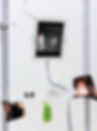

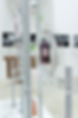
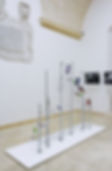